EEG
Moderated by Dr. Robert van den Berg, researcher and neurologist in training, Erasmus MC, Rotterdam, The Netherlands
Electroencephalography (EEG) for brain mapping or epileptic foci detection, involves the extracranial detection of intracranial neural activity. EEG is used as a diagnostic tool in a variety of neurological conditions, but we will limit the discussion here to applications relevant to neurosurgery.
Technical Parameters
Similar to MEG (1), the signal captured in EEG is generated mainly by postsynaptic potentials of cortical neurons. Its spatial resolution is partially determined by the number and distribution of scalp electrodes used during recording. In general, the spatial resolution of EEG (centimeters) is much less than that of MEG. MEG localizes neural activity more accurately than EEG because magnetic fields are less perturbed than electrical potentials by overlying brain structures and the skull itself (1). Dependent on the sampling frequency (in general between 250 and 1000 Hz), EEG has the potential to sample in the millisecond range, enough to monitor the underlying temporal dynamics of neuronal activity.
Biological Substrate
The signal recorded in EEG is determined by the averaging of extracellular field potentials, generated by the intracellular currents. This in contrast to MEG, where the magnetic field detectable outside the head is produced by intracellular current flow in the active neurons (1). For epilepsy surgery, the pre-operative EEG combined with video-recordings is used to support the decisions 1) whether a patient would be a candidate for surgery and 2) where the epileptic zones that need to be resected are located. Clinical literature also describes simultaneous EEG-fMRI recordings for epilepsy-related surgical planning, where the spatial resolution of MRI can be supplemented with the temporal resolution of EEG to localize seizure foci (2–4) (Figure 2). For neuro-oncological procedures, such as awake craniotomy, not much has been reported on the use of pre-operative EEG for pre-surgical mapping.
Intra-operative applicability
Conventional EEG is a purely extracranial, pre-operative tool, with Electrocorticography (ECoG) being its intracranial counterpart. In surgical planning for epilepsy, the technique has proven to be vital in accurate planning and pre-surgical decision-making due to its advantages of sampling the signal closer to the source and improving spatial resolution by avoiding skull-based signal attenuation.
One alternative consists of so-called intracranial EEG or stereotactic EEG (sEEG), where a type of Deep Brain Electrode (DBE) is implanted in the brain tissue (5) (Figure 2). These electrodes allow for more depth-resolved detection of for example epileptic zones, but they also have known applications in brain tumor surgery or functional neurosurgery (5). Although their temporal resolution remains the same, sEEG allows for a spatial resolution ranging from a micro-scale (used in for example functional neurosurgical procedures for Parkinson’s to distinguish anatomical regions based on their firing pattern signature) to the more conventional macro-scale involving Local Field Potentials (LFPs) as also measured by ECoG (see below) (5). In some cases, these sEEG electrodes are placed chronically prior to the surgical resection to allow for time to perform actual seizure detections. ECoG and sEEG registrations can also be performed simultaneously, combining measurements on superficial and deep sources.
Intravascular EEG seems the most recent, intracranial EEG alternative, where the latest generation of so-called stentrodes placed in superficial cortical veins and the superior sagittal sinus allow for recordings from the underlying sensorimotor cortex (6,7). However, variation in the exact position of the recording tip of the electrode can make source localization difficult. Here, the field does not only see potential for neurosurgical planning purposes but also for chronic applications in Brain-Machine Interfaces (BMIs) (6,7).
EEG and Open Science
Raw EEG data which is clinically acquired is often stored in a proprietary file format. The variety of different formats available in the field can be a great hindrance to collaboration, especially in reusing data or building large-scale datasets. This limits the reproducibility of results. Several attempts have been made to define open standards for EEG data, notable examples being the EDF- and its extension EDF+-file format (8). The website of this project contains a valuable overview of convertors and other tools (9). The EEG-BIDS standard is a more recent and broader attempt to create a consensus on organizing and sharing EEG data according to the FAIR principles (10).
For analysis of EEG data, several excellent and open toolboxes are available online. Examples using the MATLAB platform are the EEGLAB (12) and FieldTrip (12) toolboxes. Although MATLAB itself is commercial software, these toolboxes can also be used with the open source alternative GNU Octave, although with some limitations. Both toolboxes can be used together in the same analysis pipeline. The websites for each of these projects provide valuable tutorials and sample datasets. Similar functionality for analysis of neurophysiological data using the Python programming language is provided by the MNE library (13).
Open available large EEG datasets are sparse. Especially the sharing of clinically acquired data is complex, not only due to issues concerning privacy and consent, but also because accurate interpretation of the EEG signal is strongly dependent on a detailed description of the clinical condition of the patient during the acquisition of the EEG signal. Factors such as level of consciousness or encephalopathy, sleep-wake cycle and the use of sedative or anti-epileptic drugs should be taken into account. For pediatric patients, this is even more complex as the background pattern matures over time. A notable example of an excellent online repository is maintained by the Child Mind Institute (14). The current rapid development of machine learning and deep learning techniques and their application to neurophysiological data requires an infrastructure for open exchange of high quality, well annotated EEG-data. This is one of the most urgent challenges for the neurophysiological research field in the coming years.
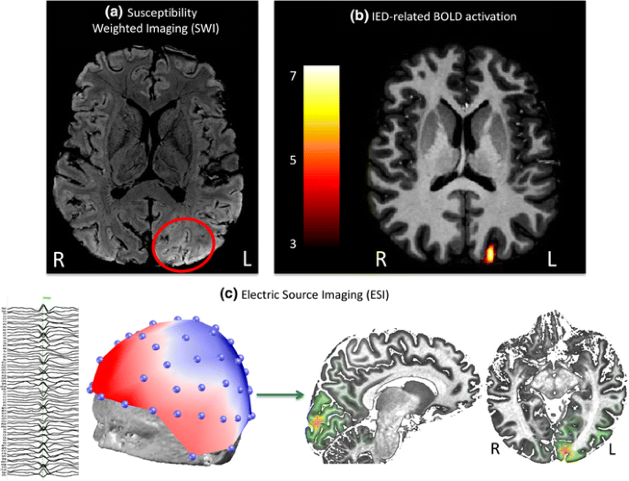
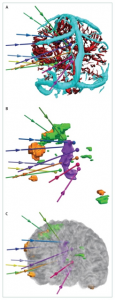
References
- Mäkelä JP, Forss N, Jääskeläinen J, Kirveskari E, Korvenoja A, Paetau R. Magnetoencephalography in neurosurgery. Neurosurgery. 2006.
- Grouiller F, Jorge J, Pittau F, van der Zwaag W, Iannotti GR, Michel CM, et al. Presurgical brain mapping in epilepsy using simultaneous EEG and functional MRI at ultra-high field: feasibility and first results. Magn Reson Mater Physics, Biol Med. 2016;29(3).
- Kekhia H, Rigolo L, Norton I, Golby AJ. Special surgical considerations for functional brain mapping. Neurosurgery Clinics of North America. 2011.
- Duncan JS, Winston GP, Koepp MJ, Ourselin S. Brain imaging in the assessment for epilepsy surgery. The Lancet Neurology. 2016.
- Lachaux JP, Rudrauf D, Kahane P. Intracranial EEG and human brain mapping. In: Journal of Physiology Paris. 2003.
- He BD, Ebrahimi M, Palafox L, Srinivasan L. Signal quality of endovascular electroencephalography. J Neural Eng. 2016;
- Sefcik RK, Opie NL, John SE, Kellner CP, Mocco J, Oxley TJ. The evolution of endovascular electroencephalography: Historical perspective and future applications. Neurosurg Focus. 2016;
- Kemp B, Olivan J. European data format “plus” (EDF+), an EDF alike standard format for the exchange of physiological data. Clinical Neurophysiology, 114(9), 1755–1761. 2003;
- https://www.edfplus.info/downloads/index.html
- Pernet CR, Appelhoff S, Gorgolewski KJ, Flandin G, Phillips C, Delorme A, Oostenveld R. EEG-BIDS, an extension to the brain imaging data structure for electroencephalography. In Scientific Data (Vol. 6, Issue 1). Nature Research. 2019;
- Delorme A, Makeig S. EEGLAB: an open source toolbox for analysis of single-trial EEG dynamics including independent component analysis. In Journal of Neuroscience Methods (Vol. 134). 2004;
- Oostenveld R, Fries P, Maris E, Schoffelen JM. FieldTrip: Open source software for advanced analysis of MEG, EEG, and invasive electrophysiological data. Computational Intelligence and Neuroscience. 2011;
- Gramfort A, Luessi M, Larson E, Engemann DA, Strohmeier D, Brodbeck C, Goj R, Jas M, Brooks T, Parkkonen L, Hämäläinen M. MEG and EEG data analysis with MNE-Python. Frontiers in Neuroscience, 7 DEC. 2013;
- http://fcon_1000.projects.nitrc.org/indi/cmi_healthy_brain_network/sharing_neuro.html